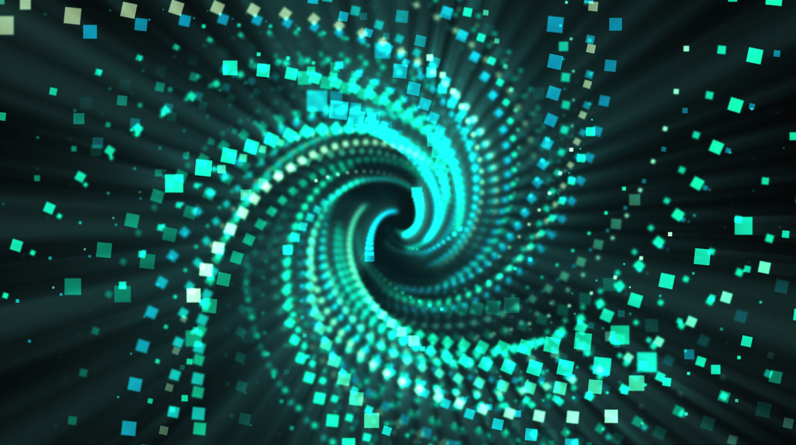
What do we mean by financial crisis? What are some of the classical methods that predict such crises? How can machine learning algorithms contribute to anticipating them?
Financial crises take a variety of forms: They range from sovereign defaults to bank runs to currency crises. What these episodes all have in common is that an internal vulnerability worsens over time and, after an associated trigger, precipitates a financial crisis.
Pinpointing the specific trigger can be difficult, so the evolution of internal vulnerabilities must be monitored. What precisely are these internal vulnerabilities? In statistical terms, they are the explanatory variables in crisis models. In historic crisis episodes, they often served as the response variable.
While this is part of the classical approach to modeling financial crises, it isn’t the only way to model financial risks.
In the classical crisis model, the standard method is to use logistic regressions to estimate the probability of a financial crisis. Explanatory variables are connected to the response variable with a non-linear link function. The dependent variable is 0 for no crisis and 1 for crisis. This approach hinges on the definition of financial crisis. The past variables are modeled with the help of maximum likelihood by varying the exposures of the explanatory variables to the response variable. In machine learning terms, this a supervised learning technique or a logistic regression with one hidden layer. It is also known as a shallow neural network.
Determining default or crisis probabilities from market prices are among the other crisis modeling methods. For example, from credit default swaps (CDS), an implied default probability can be calculated. Of course, this is fundamentally different from both the logistic regression described above and the application of machine learning algorithms described below.
So, what can machine learning algorithms do to improve on the estimation of financial crisis probabilities? First, unsupervised learning is distinct from supervised learning in that there is no response variable. Clustering is one technique that is worth highlighting. The goal of clustering is to group data points in a sensible way. These data groups will be associated with a center of mass to help determine the structure within the datasets. Clustering can be applied to both the dependent and independent variable. Rather than using a fixed threshold to determine a currency crisis, for example, we can split currency returns into different clusters and derive a sensible meaning from each cluster.
Machine learning algorithms can add significant value in this way. While clustering is only one example of the power of coding, these algorithms have a number of other useful applications
Of course, while machine learning is simply an umbrella term for many useful algorithms, whether the machine actually learns is a different question entirely.
To split the time series in a training and test set is, however, is still among machine learning’s major weaknesses. How do you determine the split? Often the decision is arbitrary.
Whatever these shortcomings, they hardly detract from the significant benefits that machine learning can bring. Indeed, now is the time to invest in these capabilities.
If you liked this post, don’t forget to subscribe to the Enterprising Investor
All posts are the opinion of the author. As such, they should not be construed as investment advice, nor do the opinions expressed necessarily reflect the views of CFA Institute or the author’s employer.
Image credit: ©Getty Images/noLimit46
Professional Learning for CFA Institute Members
CFA Institute members are empowered to self-determine and self-report professional learning (PL) credits earned, including content on Enterprising Investor. Members can record credits easily using their online PL tracker.